Simulating neurons or how to solve delay differential equations in R
I discussed earlier how the action potential of a neuron can be modelled via the Hodgkin-Huxely equations. Here I will present a simple model that describes how action potentials can be generated and propagated across neurons. The tricky bit here is that I use delay differential equations (DDE) to take into account the propagation time of the signal across the network.
My model is based on the paper: Epileptiform activity in a neocortical network: a mathematical model by F. Giannakopoulos, U. Bihler, C. Hauptmann and H. J. Luhmann. The article presents a flexible and efficient modelling framework for:- large populations with arbitrary geometry
- different synaptic connections with individual dynamic characteristics
- cell specific axonal dynamics
In its simplest form, for one self-coupled neuron or you could regard this as the synchronised case of several neurons, I have: \[ \begin{aligned} \frac{du}{dt}=&\alpha (-u(t) + q g(v(t-T)) + I) \\ \frac{dv}{dt}=&C (w(t)+v(t)-\frac{1}{3}v(t)^3) + \gamma u(t) \\ \frac{dw}{dt}=&\frac{1}{C}(a-v(t)-bw(t)) \\ g(v) = & \frac{1}{1 + \exp(-4v)} \\ \end{aligned} \] The variable u describes the total post-synaptic soma potential, which is the sum of all incoming signals v. The membrane potential v arrives via the dendrites after a time delay of T and is either exhibitory (q > 0) or inhibitory (q < 0). It is transformed from a pre to a post-synaptic signal by the monotonically increasing function g.
The membrane potential v itself is modelled by FitzHugh-Nagumo differential equations. They can be regarded as a simplified version of the higher dimensional Hodgkin-Huxely model.
The soma potential u is for \(0 \lt\alpha\ll 1\) on a much slower time scale than v. Thus, with u treated as constant we can analyse the FitzHguh-Nagumo (FHN) system. The FHN system has an interesting bifurcation behaviour, using u as a parameter. For certain values of u the system has a stable equilibrium; increase the value of u and the FHN system will go through a Hopf-bifurcation and periodic solutions with a limit cycle attractor appear (the neuron spikes).
Combining the FHN model with the soma potential u provides a model which is most fascinating. In my example the resting membrane potential slowly increases the soma potential until the FHN system goes through a Hopf-bifurcation resulting in periodic spiking (bursting), which in in itself pushes the potential u down, eventually causing the neuron to rest again.
Introducing the time delay T not only makes the model more realistic, it allows me to control the length of the bursting phase as well. A more detailed examination of the system is presented in Bursting activity in a model of a neuron with recurrent synaptic feedback by F. Giannakopoulos, C. Hauptmann and A. Zapp.
To simulate and analyse the model in R, I use the deSolve package, maintained by Thomas Petzoldt. The package comes with an excellent vignette and the DDE solverdede
. The function dede
is actually easy to use, once you get your head around it. Here is an example of the above model:
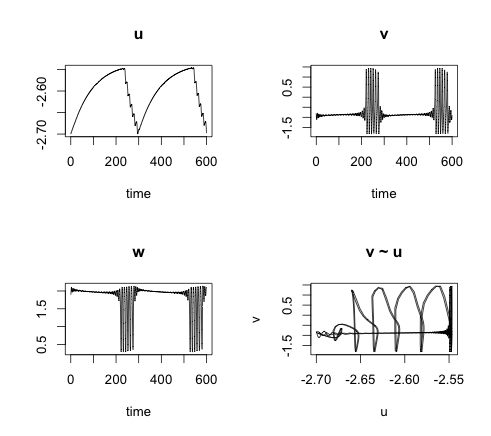
R Code
Citation
For attribution, please cite this work as:Markus Gesmann (Nov 13, 2012) Simulating neurons or how to solve delay differential equations in R. Retrieved from https://magesblog.com/post/2012-11-13-simulating-neurons-or-how-to-solve/
@misc{ 2012-simulating-neurons-or-how-to-solve-delay-differential-equations-in-r,
author = { Markus Gesmann },
title = { Simulating neurons or how to solve delay differential equations in R },
url = { https://magesblog.com/post/2012-11-13-simulating-neurons-or-how-to-solve/ },
year = { 2012 }
updated = { Nov 13, 2012 }
}